12 juni 2024, Nikki Weststeijn
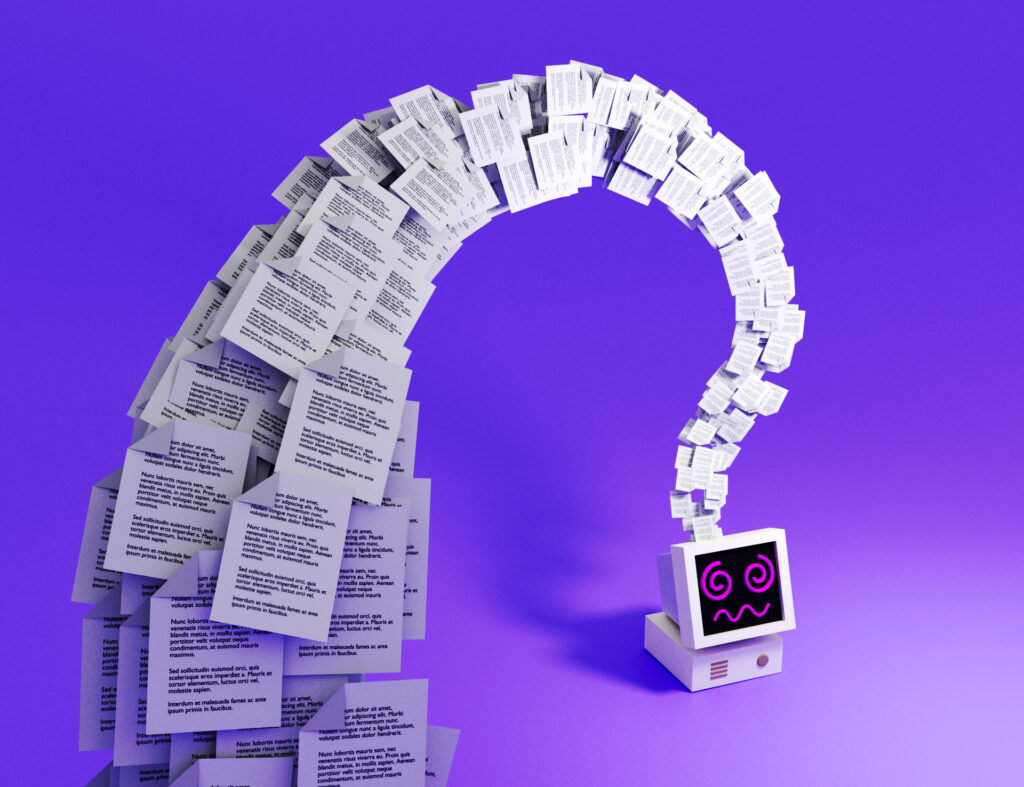
If ChatGPT gives us an answer to a factual question, we have no idea if this answer is really true. Open AI, the makers of ChatGPT, bear no responsibility that their chatbot and text generator will reply truthfully. Similarly, if an employer uses some type of language technology to screen the CV’s of applicants, there is no promise that the technology will not be biased in some way.
This is because these technologies are all self-learning algorithms. It is a type of artificial intelligence that is trained by seeing sets of exemplary inputs and correct outputs and then learns some strategy to come up with outputs on its own. It is unclear why the algorithm will give one answer and not the other.
Floris Roelofsen, professor at the ILLC, is director of a new large-scale research project called ‘Language Sciences for Social Good’ (LSG) at the University of Amsterdam. The idea behind this overarching research project is to make sure that language technology will also be used for social good, and not just guided by cost-efficiency and result-driven goals. Roelofsen: “Language technology is booming, it’s really transforming society. With ChatGPT it’s very clear, but this development has been going on for the past 25 years. We have been using language technology when we search on google, when we translate pieces of text, or when we use voice assistants on our phone.”
Language technology has a lot of impact, but in areas where it could potentially have a positive impact, it is still lacking, according to Roelofsen. The LSG project has a threefold goal: to use more responsible methods in developing language technology, and make sure that language technology can contribute to a safe society and an inclusive society.
One of the main goals in terms of using responsible methods in language sciences, is that language technology should be more transparent. Going back to the CV-screening example: in the training phase, the algorithm is shown sets of CV’s and the cv that in the past has actually been picked by an employer. The algorithm then learns a way to select a CV out of a pile of CV’s, the one that is supposed to belong to the best candidate for the job.
Unless we add additional rules to the program, this algorithm will not make the decision in a way that is similar to how a human makes the decision. It is not a decision based on certain principles, like the fact that the candidate needs to be trustworthy, hardworking and smart. It will just be a decision based on what is the most likely outcome given the examples that it has seen.
A researcher at the ILLC who has started doing research related to the transparency of AI is Fausto Carcassi. He is a cognitive scientist and is working on a model that uses probabilities to determine the meaning of a word in different contexts. Carcassi hopes that methods of this model can also be used by Large Language Models (LLM’s) such as ChatGPT and that, vice versa, techniques used by LLM’s can also improve the model he is working on.
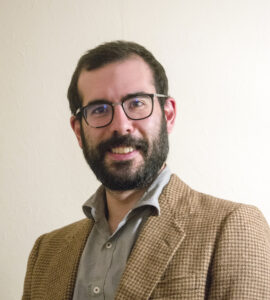
If you read an answer given by ChatGPT, the language used seems very human. Yet, there is no human behind the screen that actually understands the answer that is given. We also don’t really know how the algorithm knows what to answer, because it has learnt in its own way when it should give a certain output. In this sense, algorithms like this are considered ‘uninterpretable’ or ‘non-transparent’.
Fausto Carcassi is a cognitive scientist who studies how the meaning of words and phrases changes in different contexts, which is known as ‘pragmatics’. He is trying to find a way to combine his pragmatics model with the techniques used by large language models like ChatGPT.
By building some of the methods developed in his pragmatics into large language models (LLM’s), he hopes that it will become more understandable why an LLM will give one answer in a certain context and not in another. Carcassi: “With these large language models, it’s like they know how to draw a plant, but they don’t even know what a plant looks like.” Possibly, it can also make AI give answers that are better suited to the context and, for example, not offend anyone who is reading the answer.
The pragmatics model Carcassi is working with is known as the ‘Rational Speech Act model’ (RSA). It is a model that determines the meaning of words and phrases in different contexts based on probabilities. Even though words have a standard, literal meaning, some utterances also change meaning dependent on the situation. The word “I” for example, has a different meaning depending on who uses the word, who is being referred to. Similarly, the phrase “OMG” can be used to express frustration, but it can also be used to express relief.
The RSA model defines the likelihood that an expression has a certain meaning. For example, in some context, it could give a 40% probability to the fact that a speaker used “OMG” to express frustration and a 60% probability to the fact that the speaker used it to express relief.
The model can also be used to determine what utterance is best to use for a speaker in a certain situation, if they want to solve a specific task. For example, if there is a blue square, a blue circle and a green square, and the speaker has to refer to the blue circle, using a single property. The easiest way to do so is refer to it as “the circle”. If it would have referred to it as “the blue thing”, a listener would not be able to know if the speaker was referring to the blue circle or the blue square.
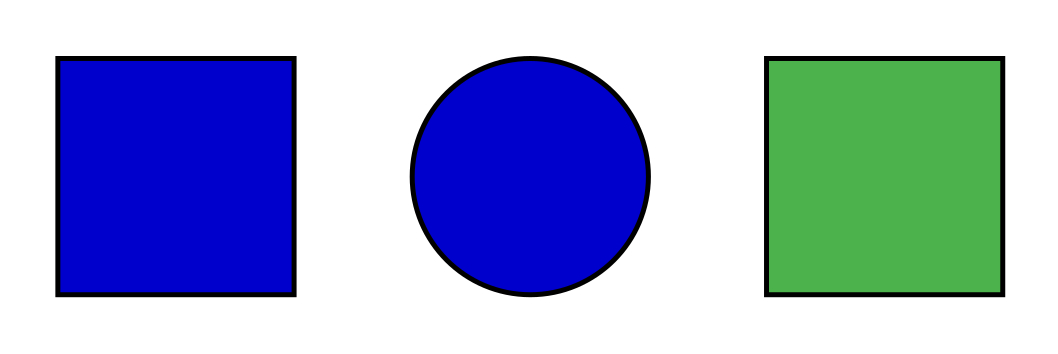
RSA has been tested in experimental settings and has been shown to accurately predict how humans use language and understand language in a given context. However, RSA has only been developed and tested for very simple scenario’s, such as with the green and blue circles. A situation in which an expression like “OMG” is used is now still too complex. One of the goals with this project is to incorporate some of the techniques used by LLM’s into RSA models, so that it can become more open-ended and deal with more situations. Carcassi: “Hopefully, we can to some extent shrink down the gap between these scientific models and real-world language use.”
The next step would be to see if some of these methods can be built in to AI. The idea is that a large language model like ChatGPT could, given a certain context, come up with a few possible answers to give to a user. Using RSA-methods, it could then determine which answer is best to give. This would make the model more interpretable. A programmer would, for example, be able to see the options between which the model pickes. And we would know why the model would select an answer.
Using RSA methods in LLM’s would also provide the possibility to build in certain principles, like politeness, that the model has to consider when deciding between different options. Carcassi: “So, in developing RSA, we also want to look specifically at more complex social dynamics. What are the rational strategies people use to strike a balance between communicating information and not hurting the other person?”
By first understanding how humans do this, the hope is to also be able to implement these strategies into AI. Carcassi: “This shows the importance of linguistics: if we don’t understand it in humans, how could we ever establish it in computational models.”
Carcassi’s research is an example of how creating more transparent AI could also contribute to developing safer AI. Safety of AI is a big concern. Language technology can be harmful on different levels. It can offend people, for example by producing a sexist poem. If used to screen cv’s, it could discriminate certain social groups, for example by picking out white men more often. A chatbot like ChatGPT can also be directly endangering, for example by providing instructions on how to build a bomb upon request.
Ekaterina Shutova, Associate Professor of Natural Language Processing at the ILLC, does research on how we can prevent technologies like ChatGPT from producing hate speech or otherwise discriminatory content. She recently received a grant from Meta, the company that owns Facebook and Instagram, to do similar research on misinformation.
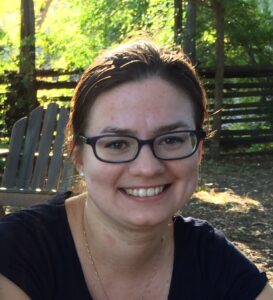
We can ask ChatGPT to translate something for us, we can ask for some factual information, we can even ask it to write an entire essay or a poem. But what if the chatbot creates a poem that is extremely sexist? Or if we ask it to predict who is most likely to commit a crime and it will give a biased answer?
ChatGPT has built-in filters that are supposed to make sure that it does not generate harmful answers or content. One of the ways in which ChatGPT is trained to create safe content is by learning with human feedback. After the initial training phase, the model is tested by a human who can tell the algorithm when the language produced is inappropriate.
However, ChatGPT could still be provoked to make hateful comments. If the systems parameters are for example set such that the chatbot should behave like a historical figure, like Donald Trump, the outputs can be much more hateful. Users can also try to find clever ways to prompt the chatbot in such a way that it creates a hateful comment.
If a language model that is used in decision making is biased against certain social groups, this could also have bigger consequences. Shutova: “If we actually want to use these types of algorithms in decision making, for example for recruitement or for medical diagnosis, then we need to systematically understand what kinds of biases these models show.
One of the lines of research supervised by Shutova is to see if these biases exist and then to find the sources of the bias. Together with her PhD students, Shutova analyzes what stereotypical properties a language model associates with certain social groups and if there are certain emotions, such as fear or anger, associated with that group, for example with immigrants. Shutova: “And you see that there are really no surprises there: the AI mimics the biases that already exist in society.”
The next step is to find a way to decrease the amount of implicit bias in a model. One possible way is to first look at the training data and filter out data examples that have offensive language in them. But this is harder when we want to prevent complex implicit biases. Shutova: “With explicitly offensive language, you can just filter it out. And everybody agrees that we wouldn’t want to have that. But a lot of things are really subtle. It’s really how you talk about a social group in lots of different ways across lots of different documents.”
Shutova and her student Vera Neplenbroek are therefore working on a different approach. This is to try to get rid of implicit biases after the model has had its initial training. This method tracks what specific examples in the training data caused the model to inherit a certain bias and then omits these examples at a later training stage. Shutova: “We have shown that omitting these examples during training really helps to reduce the bias. So, we hope that this is an approach that would be picked up.”
A problem with most of the existing debiasing techniques is that it is focused mostly on gender bias. Shutova’s method is designed to work for eliminating different kinds of biases, including gender bias, but also biases that are related to your level of education or economic status for example.
Shutova is working on a method to take out bias áfter the model is already designed and trained. This is because, at the moment, most companies that develop AI still consider safety to be an issue that should be dealt with after the technology is developed. However, AI can in principle also be developed with safety as one of the main requirements.
One could even develop AI with positive goals in mind, such as to make society more inclusive. Roelofsen: “The development of language technology is now mostly driven by commercial interests, even though there is so much potential to design things that can be good for society. Universities are not commercial. And I believe that it would be a waste if we would be doing the same things commercial companies are already doing.”
An example of ways in which language technology can contribute to a more inclusive society is the sign language project that Roelofsen has been working on for a few years. One of the aims of this project is to create an avatar that can produce sign language. This is much needed according to Roelofsen, because currently deaf people are in many ways excluded from society. Roelofsen has a young daughter who is born deaf and has noticed that Dutch sign language (NGT) is not really taken seriously as a language. “Currently, in the Netherlands the consensus seems to be that when a child is born deaf, it should learn to speak Dutch. But this is much harder when you cannot perceive the sounds. It’s like having to learn Chinese while never being able to actually listen to it.”
According to Roelofsen, spoken Dutch will always be like a second language to deaf people. It costs way more effort to understand, even if it is written down. Since spoken Dutch is used everywhere, it can be harder for deaf people to participate in society. This is why Roelofsen wants to create an avatar that can communicate with deaf people in their own language, for example in public spaces such as train stations. As part of this sign language project, Jos Ritmeester, a linguist at the ILLC, is working on an app that can help parents of deaf children learn sign language.
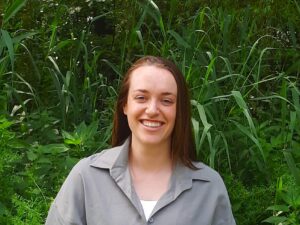
More than 90% of deaf children are born to hearing parents who do not speak Dutch sign language. Roelofsen noticed that parents are often not recommended to start learning sign language themselves and to teach it to their child. He and his partner were even advised against doing so. “The people from the organizations that are supposed to help the parents often do not even speak sign language. It was also practically impossible to find a course we could take to learn some basic words that we could teach our daughter.”
Ritmeester explains that doctors often recommend giving the child a cochlear implant. This is a surgically implanted device that stimulates the auditory nerve in the brain and thereby allows deaf children to hear. According to Ritmeester, such implants do not always have the right outcome. “This implant does not allow deaf people to hear in the same way as hearing people. It costs a lot of energy to filter out noise. There can also be complications with the implant, it can for example cause bad headaches.”
This can be a reason for people to take out the implant. “And then, if they did not learn sign language, they are lost.” According to Ritmeester, there are now a lot of studies being done on the consequences of not learning sign language as a deaf or hearing-impaired child. It seems that it can have a negative impact both on the cognitive and social development of a child.
In light of all of this, Roelofsen wanted to create an app that can help parents in the Netherlands learn Dutch sign language (NGT). The app is meant to be complementary to actual lessons that parents can follow in person. Parents should be able to use the app in their spare time, or if they want to double check something. The app is supposed to fit in with the education programs provided by Auris, an organization that provides services and education to deaf people.
The idea is that parents can look up certain phrases or sentences, and that it will show videos of a person performing the sign language translation. The videos will also have subtitles that show the literal translation to spoken language.
Currently, there is an online dictionary for NGT, but it does not explain how you can make full sentences. It also does not include explanations of the grammar. Ritmeester: “Because sign language also has grammar. For example, if I say in sign language that I give something to you, it will look very different then if I say that I will give something to them. The verb really conjugates.” The app will therefore also include grammar tutorials, equally taught in sign language.
Ritmeester’s project is an example of one of the ways in which language technology can help people who speak a minority language feel more included in society. In general, the large language models that are developed now, are trained mostly on English texts. Simply because these models need lots of data and English texts are widely available. These models cannot speak languages that are used by fewer people in the world. Such as sign languages for example.
But it is exactly for these types of languages that language technology could really play an important role. For immigrants in the Netherlands who do not speak Dutch, for example, it is much harder to decide who to vote for, to understand government programs like the vaccination programs during the Covid pandemic, or to defend themselves in court. Language technology could help these people to become more included in society. Roelofsen: “It can really make a difference for some people.”