11 October 2021, Iris Proff
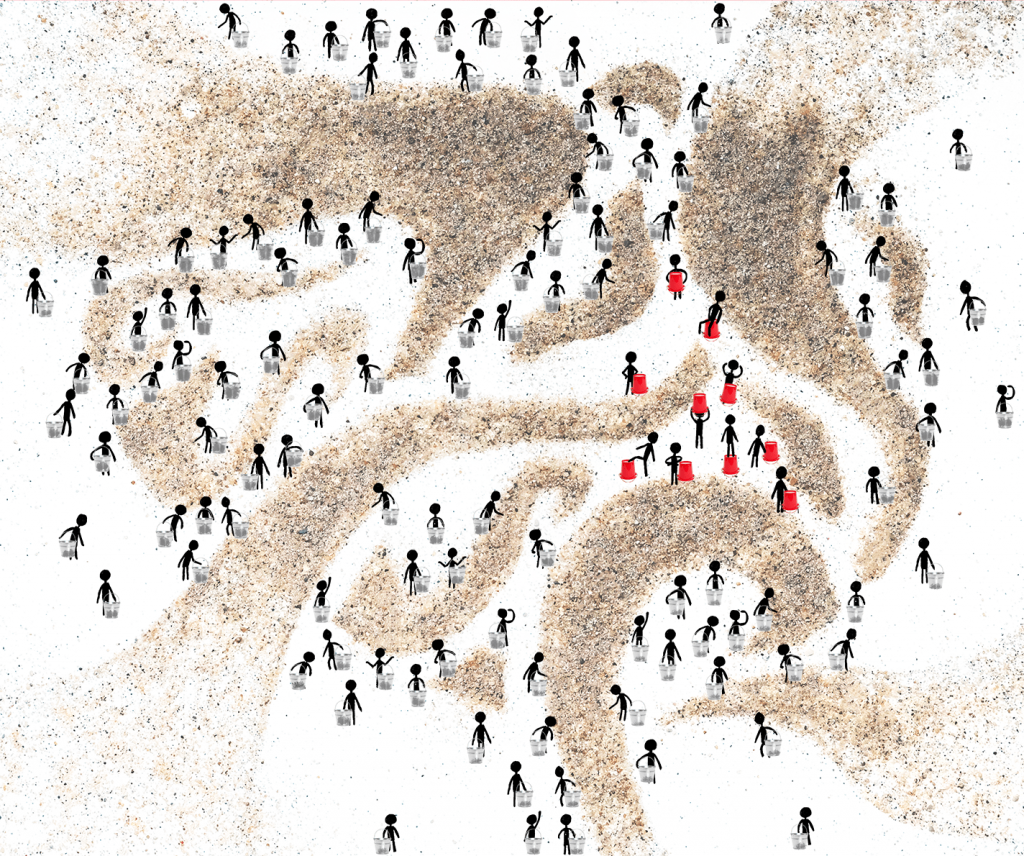
The hive mind, swarm intelligence or the wisdom of the crowds – there are many expressions for the simple intuition that groups are better at solving problems than individuals are. Flocks of migrating birds manage to find their route between breeding and wintering grounds over thousands of kilometers year after year. Ant colonies can identify the optimal path to a food source. And when a large group of people is asked to guess the weight of a bucket of sand, their averaged guesses can be pretty close to the true weight.
A theorem of collective wisdom
In each of these somewhat mysterious cases, individual guesses might be quite far off. However, the errors individuals make balance each other out, such that the collective arrives close to the “truth”. This idea was formalized by the 18th century statesman, mathematician and philosopher Nicolas de Condorcet in what has come to be known as Condorcet Jury Theorem. Roughly speaking, it states that groups are better than individuals at identifying the truth, and that they get better and better as the group gets larger.
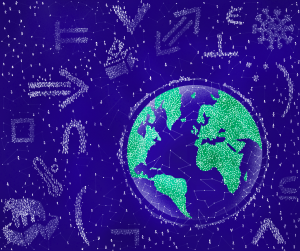
The Condorcet Jury Theorem and its many extensions and implications are one of the interests of Adrian Haret, researcher in the Computational Social Choice group at the ILLC. “The theorem holds only under very special, somewhat unrealistic conditions”, says Haret. One of those is that individual guesses must be independent. In other words: the agents, be it birds, ants or humans, are not allowed to influence each other. That might be true for artificial guessing games where people quietly note down their beliefs. But in reality, we are very much influenced by other people’s beliefs in our search for truth. When it goes wrong, this interaction can break the wisdom of the crowds and lead to polarization of opinion, informational cascades, and filter bubbles. But when it goes right, interaction might actually increase people’s ability to find truth.
Prediction markets as crystal balls
For a powerful example, let’s turn to a rather painful chapter of the recent history of empirical research: the replication crisis in Psychology. Between 2012 and 2014, a group of 92 researchers placed bets on which results from 44 published psychological studies would be replicated in independent repetitions of the original experiments. They did so in two different manners: First, they quietly noted down their own beliefs. Then, they participated in a prediction market, where they could trade contracts for different studies with each other, similar to assets on a stock market.
Interestingly, the silent guesses of the researchers were not predictive of which results would later be replicated. The prices of the contracts after trading however indicated with 70 percent accuracy which results would be replicated (rather shockingly, more than half would fail to replicate). Only by trading contracts back and forth and by adjusting their beliefs to what other people think could the researchers collectively arrive at a consensus and get closer to the truth.
A simple model of social learning
In the 1970s, the American statistician Morris DeGroot built a mathematical model of how such a consensus can arise out of interaction between individuals. The now famous DeGroot model consists in a network of agents that start with an initial belief about a certain variable. This variable might represent the weight of a bucket of sand or the mortality rate of a viral disease. Step by step, they update their belief by replacing it with a weighted average of some of the other agents’ beliefs – those they are connected to in the network.
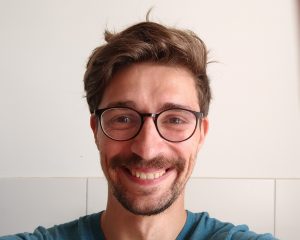
DeGroot found that in this simplistic model, all agents’ beliefs converge to one and the same belief over time. This is remarkable because “there isn’t any central authority that has access to all the beliefs”, says Adrian Haret. “It’s just the agents updating locally, and somehow they manage to reach the same result as if they were to pool all their beliefs.”
It wasn’t until 2010 that Benjamin Golub and Matthew O. Jackson added the notion of truth to the DeGroot model. They showed that if the agents’ beliefs are centered around a “true” value and the group is large enough, then, by repeatedly updating their beliefs, they will eventually converge not just to any value, but to the truth. However, Golub and Jackson found that this truth finding only works if the network is structured in a certain way: As the network grows larger and larger, every agent’s influence must diminish, and eventually vanish. If there is a prominent individual or group that, in virtue of its privileged position, exercises an outsized influence on what the group believes, this will prevent the group from finding the truth.
Pure or oversimplified?
The DeGroot model – also known as naïve social learning – can capture the basic principle underlying mysterious wisdom of the crowds effects such as prediction markets. “It’s a very purified view of the flow of information in a social network,” says Adrian Haret. As such, it is heavily simplified compared to real-world social learning. How so? First, it requires every agent to trust the information obtained from other agents and to take it into account when forming a new belief. It thus ignores the fact that real people might be selective about which information to trust and do not easily throw away their prior beliefs when new information comes in.
Second, when the agents update their beliefs, they rely solely on information from other agents and do not have any contact with the external world. The model thus assumes, in a way, that each individual is born with a certain belief about the mortality rate of a disease, which they will subsequently forget and replace by whatever other individuals around them believe.
Misinformation kills social learning
Despite these somewhat absurd simplifications, the DeGroot model allows researchers to explore what happens when the basic mechanism of social learning is distorted. For instance, a group of researchers from Tel Aviv recently investigated the effect of non-cooperative agents who forever stick with their initial belief and never update it. These non-cooperative agents, dubbed “bots” by the authors, mimic misinformation spreaders in a social network. The research team found that with a single bot in the network, all other agents eventually adopt that bot’s belief. While certainly exaggerated compared to a real-world scenario, this suggests that social learning is highly vulnerable to persistent misinformation.
A new, more cautious learning rule proposed by the authors can prevent the catastrophic effect of bots. Agents following this rule change their belief maximally to a certain extent and only if the evidence speaks strongly against it. While this renders learning resistant against bots, it also prevents the agents from finding a perfect consensus – instead their opinions end up being scattered around the true value.
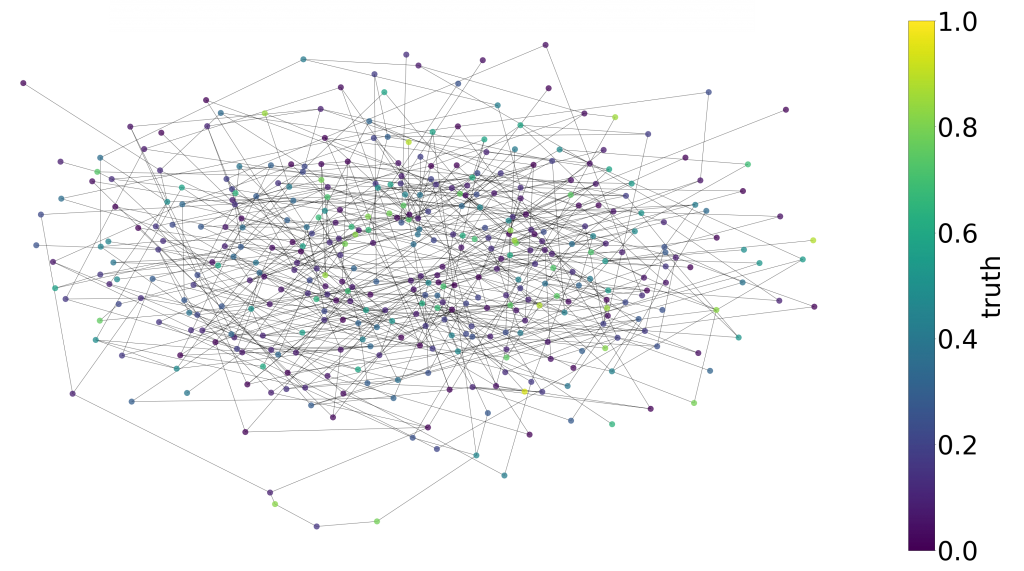
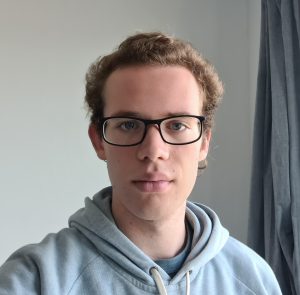
In his Bachelor thesis at the ILLC, Adrian Haret’s student Roman Oort replicated this finding and found that another updating rule, the so-called “private belief rule” has a similar effect. Here, besides considering beliefs of other agents, an individual always hangs on to their initial and fixed “private belief” – think of it as a first gut feeling, or anchor for future beliefs – and includes this private belief in every further update. Both approaches essentially “make the agents more sceptical, or stubborn”, says Roman Oort. A healthy amount of scepticism – or stubbornness – might also help people in the real world resist the effects of persistent misinformation, the student argues. “There is a sweet spot between having very trustful and very suspicious agents that is incredibly hard to find”, says Adrian Haret.
All models are wrong?
The elephant-in-the-room-question remains: Can mathematical models of social learning teach us something about the real world? Variants of this question apply to modeling research in all kinds of disciplines, from modeling the climate, to modeling the economy or modeling the brain. Social learning – just as the climate, the economy, and the brain – is a complex process with countless variables that play into it. Any mathematical model of social learning will rely on heavy simplifications . This means that effects observed in learning models do not translate one-to-one to reality.
However, social learning models allow us to think systematically about how things like misinformation or highly prominent agents affect the basic mechanism of social learning. They allow us to identify under which network structures filter bubbles or informational cascades can emerge. And they allow us to make careful suggestions about how agents should behave or how the network should be structured to resist such distortions. It all comes down to the famous quote by the British statistician George Box: “All models are wrong, but some are useful.”