November 22 2024, Alma Apt
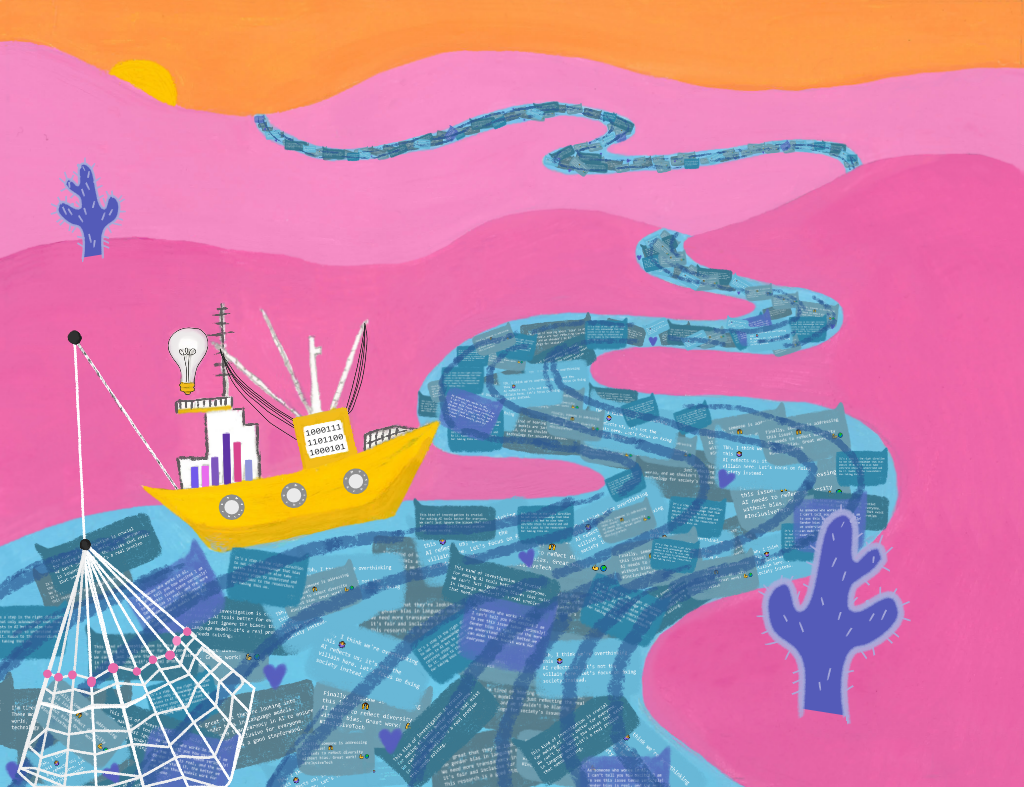
These novel scientific possibilities have resulted in a growth spurt of new disciplines, such as Digital Humanities, Computational Sociology and Social Complexity Theory. Computational Social Science (CSS) is currently becoming an umbrella term for many approaches that bring together computational methods and social science questions, utilising mass digital data for scientific research.
With a new bachelor’s of Computational Social Science at the UvA and two new UDs in the topic at the ILLC, drs. Petter Törnberg and Roberto Cerina, CSS has become a rapidly expanding discipline at the university. I sat down with Petter and Roberto to discuss the emergence of CSS as a field, their own work within it and the place CSS takes within the ILLC.
What is computational social science?
The term ‘Computational Social Science’ may seem, at first glance, somewhat ambiguous. It announces its method—computation—and its area of application—social science. However, unlike disciplines like Computational Sociology, CSS doesn’t align itself with a specific academic tradition or disciplinary framework. Instead, it’s an umbrella term, encompassing various methodological and theoretical influences. Interdisciplinarity is inherent to the field.
One reason for the openness towards a wide variety of influences, as Roberto explains, is that CSS is driven by technological developments rather than theoretical traditions: “I think CSS really comes from the technology first. It’s not like econometrics, which starts with formal modeling and then applies that through statistical assumptions. CSS is different. We have all this new data, all these new methods. The question is: What can we learn from these new resources?”
One important way in which CSS differs from traditional social science, is that established research methods often cannot be applied to digital data. Petter points out: “Getting a random sample is hopeless when you work with digital data. Nothing is independent, everything is connected, and we don’t really have rich attribute data. It’s mostly interactional.” He continues, “The assumptions that come from classical statistics just don’t fit. So we have to develop entirely new approaches. Many of these methods, like natural language processing or social network analysis, come from tech industries like Silicon Valley, not from social science. This means that we often end up post hoc applying social science theory to methods that weren’t originally designed for those purposes.”
Since CSS draws on methods from computer science, sociology, political science, and economics, the field does not have a unified body of theory. But Roberto argues that computational methods have shown how traditional disciplinary boundaries are far more blurred than institutionally assumed. “Quantitative studies done by political scientists or sociologists are often studying the same phenomena,” he explains. “When it comes to methods, you often can’t tell them apart. CSS cuts through these barriers, creating a space for people who are excited about using computational methods to study social phenomena.”
Petter agrees, noting that CSS is both methodologically and theoretically pluralist. “We tend to approach theory in a way that’s more like, ‘These are tools we can use to tell this kind of research story.’ We pull from different theoretical traditions, and combine them with a range of methods to tell stories that are interesting to specific disciplines but also to a broader audience.”
This pluralism allows CSS researchers to publish across a range of disciplines and reach audiences beyond traditional academic fields. As Roberto notes, “CSS doesn’t yet have a core body of theory, partly because it’s so new, but also because the boundaries between disciplines often don’t make sense when viewed from a computational perspective.”
The Impact of Large Language Models
The rise of Large Language Models (LLMs) has had a profound impact on CSS, enabling researchers to analyze not just the structures of social networks, but also the content of communication within those networks. The ability to engage with text in a meaningful way represents a significant shift. Petter notes that “CSS used to look at the world primarily through the lens of social network analysis and complex networks. We looked at the structures of interaction and threw out the content. That approach is limited because it treats social cohesion and network cohesion as the same thing.”
Taking an example from on of his own topics of research, Petter explains the way echo chambers and radicalisation were studied in the past: If researchers identified a split in a network, they concluded the existence of an echo chamber. But this approach is clearly limited. What’s missing is the content of what’s actually being said within those networks and in-group communication doesn’t necessarily indicate the existence of an echo chamber. Petter explains that the advent of LLMs has shifted this focus: “Now we’re moving from social structures to content—text, culture, and meaning. These are all qualitative aspects we’ve been bad at analyzing in CSS so far, but with LLMs, we can now do powerful studies on them.” This, he argues, marks a fundamental shift in the discipline: “We can now measure things like polarisation not just as a network structure but as a discourse, as a latent sense of identity. That’s really exciting.”
Roberto agrees, adding that LLMs make certain types of research more practically achievable: “You can now create synthetic agents,” he says. “Before, I had to pay a lot of money to get people into a lab for experiments. Now, I can create synthetic people and run simulations. I can create synthetic social networks and study them computationally.”
While both Petter and Roberto operate within the field of CSS, their individual research focuses illustrate the diversity of the discipline. Petter calls his approach heterodox; rooted in media studies and critical theory, he examines how digital capitalism, platform capitalism, and surveillance capitalism influence the kinds of data CSS work with. “I take a reflexive stance,” Petter explains, “where I view the data itself as a form of capital, as embroiled in broader systems of inequality and so on. I’m seeing the kinds of methods that we’re using as quite limited – useful but limited – and highlighting what they’re not bringing into view”.
Roberto, on the other hand is more focused on methodology: “One of the criticisms of CSS is that we’re all starry-eyed about new data, new methods, new technologies, and that we apply these mindlessly to whatever stream of data we have and extract something. And yes, it happens, but it doesn’t mean that that cannot yield novel insights.” Roberto therefore focuses on how one can critically examine these results by reinserting statistics into CSS: “Yes, we have this new deluge of data, but is more data always better? Just because we have endless streams of data coming from social media, that doesn’t mean that they don’t represent a specific population. Is that the population that we want to study?” He specifies: “The silly thing that people say is: Can we put error bars around the estimates that we make? Which, from a statistical perspective, means: Can we make that inference? Can we reason for that inference within the context of a specific population that we’re trying to estimate? And how do the data that we observe differ from that and do we have a theory that connects the two things?’
CSS in society
As much as CSS has allowed for new inroads for scientific research, the availability of mass digital data has come at a cost. Digital privacy, data security and AI’s role in society are all politically contested topics that are still subject of changing legislation, as politics tries to keep up with the evolving digital landscape. CSS researchers have to take these questions into account, even while using this data themselves. Roberto argues that “CSS forces us to ask: What do we do with all this data? How do we handle issues of privacy? How do we ensure that AI and computational methods are used responsibly in the study of human behavior?”
Yet one criticism that has been leveled at CSS, is that its overly close relationship to Silicon Valley – which created the exact technologies CSS studies – problematises CSS’ ability to have the critical distance needed for these questions. Petter draws an analogy to economics in the 1970s: “To some degree, CSS plays the role in contemporary society that neoclassical economics played to neoliberalism, in terms of legitimizing a certain ideology. It’s part of the ideological construction of contemporary digital capitalism.”
While Roberto agrees, he adds that “it is also true that Silicon Valley has the capital and the willingness to invest in this dynamic kind of science. You need a dynamic system to be able to put in resources and believe in such a project. And Silicon Valley has this sort of creative destruction mindset that means they’re willing to do that. And other institutions – I’m thinking especially about the government and public sector – that could equally benefit from CSS tend to be more reluctant about it.”
Petter concludes: “Ultimately, even if we want to take a critical stance towards the kind of structures and ideologies of contemporary capitalism, abandoning powerful methods because they’re associated with a particular ideology is not very strategic or productive.”